Global food markets are inter-connected
Standing in a supermarket in Potsdam, food seems very abundant, and only the odd empty shelf of sunflower oil in March 2022 was a stark reminder of the interconnectedness of global food markets and the dependence on food imports. The reason for the empty shelves was that people realized that Germany imports 94% of its sunflower oil, and that Ukraine and Russia belong to the largest exporters. As there was no actual shortage of oil in Germany at the end, this was a classic example of the now infamous hoarding behaviour of some German consumers. Still, suddenly our dependence on agricultural production and the impact that local events can have on global markets became front page on German newspapers.
While empty shelves in a German supermarket are for the majority of people at most an inconvenience, almost 10% of the global population are estimated to be food insecure, and local production shocks can have far-reaching consequences. An estimated two billion people depend on small-scale agriculture and are particularly vulnerable to climatic hazards. This is a pressing issue, as extreme climate impact events are already becoming more common, and are projected to increase further.
Crop models in climate impact research
Crop models (global gridded crop models (GGCMs) as they are called in full) are an important part of climate impact research and are used to estimate the impact that rising levels of CO2 and temperature have on agricultural yields. Different studies have evaluated the performance of crop models and have shown that crop models can perform well, but that there are differences in performance between countries, crops and models (Frieler et al. 2017; Müller et al. 2017, 2019 and corresponding ISIpedia article). One unresolved question is whether this is because models do not adequately represent how crops respond to heat stress or drought conditions. Or whether the problem is rather that the crops cannot adequately represent management of crops in the form of planting and harvest dates. Concerning the response to heat, an earlier study has shown that crop models can reproduce the decline in maize and soy yields caused by high temperatures in the US ( Bernhard Schauberger’s ISIpedia aricle). Concerning management, Jägermeyer & Frieler showed that one crop model (called LPJmL) can better represent droughts and heatwaves when the length of the growing season is made more realistic.
How we evaluated the ISIMIP2a crop models
In this study, we evaluated together with colleagues, how well the models that were contributed by 13 modelling groups to ISIMIP simulation round 2a (ISIMIP2a), can represent droughts and heatwaves. To achieve this, we used data from the FAO (Food and Agriculture Organization of the United Nations) as observational data. These data are based on agricultural production and harvested area reported by countries. For the analysis, we used annual yield time-series from model simulations which we compared to the observed FAO data. In the past decades, yields have increased due to technical progress, but this is not necessarily captured by crop models. That is why we first divided each time-series by the temporal trend. The result is a unitless composite of yield change relative to the expected yield and values range around 1. This means that for values larger than one the yield was larger than the long term mean yield, and smaller for values below one. This approach has the advantage that a small yield decline in a country like Burkina Faso with much lower yields, that can still have a very strong impact locally, is represented similarly to a large yield decline in a high-yield country such as France.
To know in which years droughts or heatwaves occurred in which country, we used data from the Emergency Events Database (EM-DAT, CRED 2020). The database records all events in which more than 10 people died, more than 100 people were affected, a state of emergency was declared or there was a call for international assistance. For each drought or heatwave event we took the yield for the year of the event and the yield for the three years before and the three years after the event, resulting in a total of seven years. We did this for all events that occurred in one of the 20 largest producer countries. We then calculated the mean yield across all events in all of the 20 countries, and this corresponds to the line shown in Figures 1 and 2. The analysis was implemented for four crops: maize, rice, soy and wheat.
Models detect the impact of extreme events but mostly underestimate their magnitude
We found that under droughts yield declined in the observational data on average by 4.3% for maize, and 6.7% for wheat. For heatwaves there was a decline of 8.3% for maize, and 4.5% for wheat. Comparing this to the decline simulated by the models, we can conclude that the majority of models detected the event signal. That means that for the majority of simulations there was a yield decline for the year of the event. However, models typically underestimated the magnitude of yield decline in years with an extreme event. There were differences between models, and between crops.
Maize vulnerability to drought and heatwaves
Impact of events on maize yields averaged across all events (n is the number of events). Results are shown for the best simulation by the model (coloured line) compared to observed impacts (black line). Values larger than one mean that the yield was larger than the long-term mean yield, and values below one mean yield was smaller than long-term mean. The black line differs between plots because model runs cover different time periods and thus a different number of extreme events. The shaded area is the standard error. (hint: select a different model and extreme event using the drop-down menus)
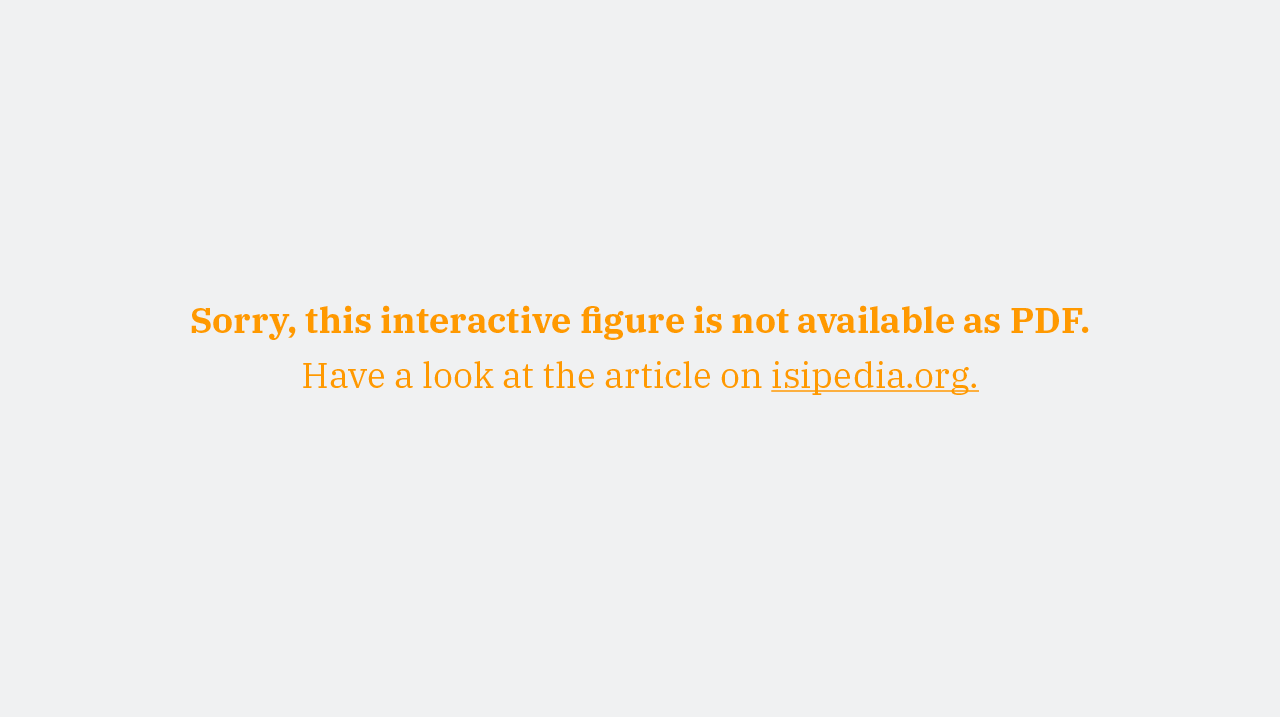
FIG 1
Models performed particularly well for maize and wheat, where 12 of the 13 models detected the event signal for maize and drought; and 11 models detected it for maize and heatwaves (Figure 1). For wheat, all models detected the event signal for droughts, but only six models detected the signal for heatwaves (Figure 2). Most models underestimated the magnitude of yield decline (Figure 1 and 2), and only one model overestimated yield decline for wheat for both event types. Details on model performance for the other two crops, rice and soy, can be found in the original publication. There was not a single model that outperformed all others. Some models were very close to observed yield decline for certain combinations of crop and extreme event type, but not for others.
Wheat vulnerability to drought and heatwaves
Impact of events on wheat yields averaged across all events (n is the number of events). Results are shown for the best simulation by the model (coloured line) compared to observed impacts (black line). Values larger than one mean that the yield was larger than the long-term mean yield, and values below one mean yield was smaller than long-term mean. The black line differs between plots because model runs cover different time periods and thus a different number of extreme events. The shaded area is the standard error. (hint: select a different model and extreme event using the drop-down menus)
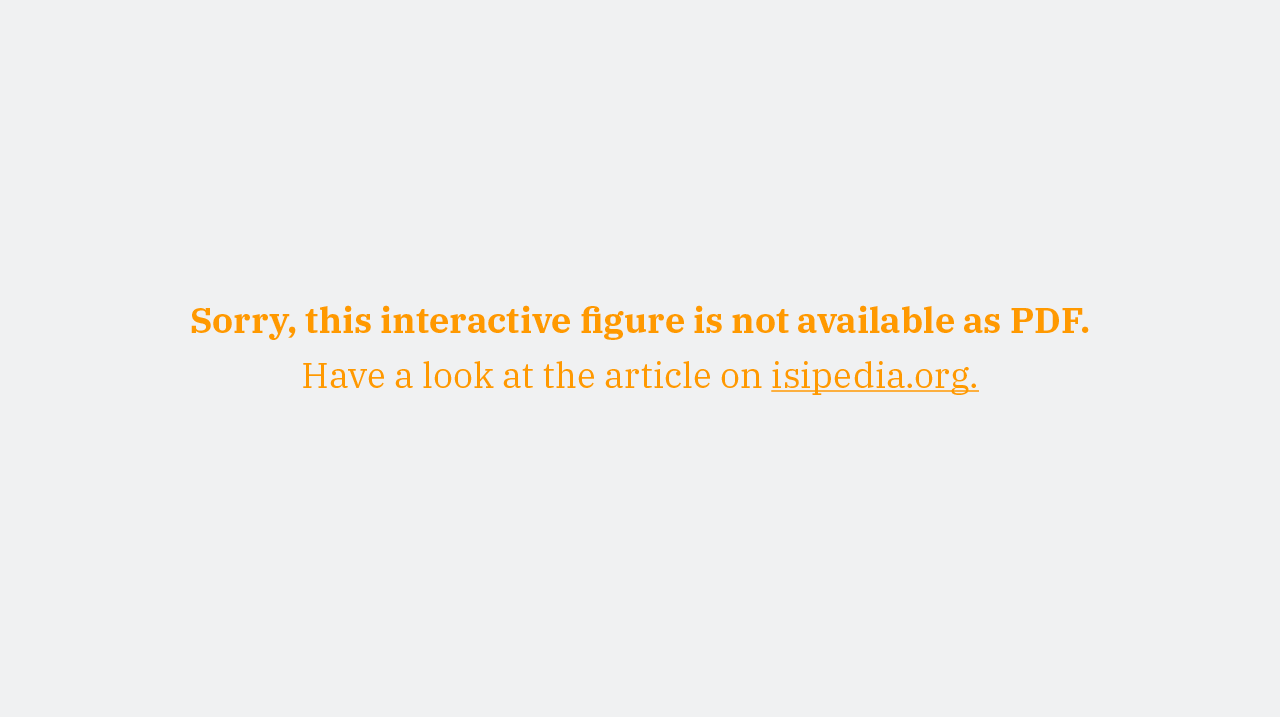
FIG 2
The follow-up question – why?
As alluded to earlier, two mechanisms could explain why there is a disagreement between observed and simulated yield decline. Either the response of a crop to heat and drought is misrepresented in the model, or the timing of the growing season is misrepresented. The latter could mean that, for example, exposure to heat or drought could occur when a crop is at a different phenological stage than observed in the real world (e.g., heat and drought could have a different impact on a plant that is still flowering versus when fruits are already developing). Or an extreme event could occur when the growing season in the model has already ended (i.e., the model “does not see” the extreme event). To test whether the timing of the growing season had an effect on model performance, we extracted the mean precipitation and number of extreme degree days (EDDs) for the simulated growing season. EDDs are those days with a maximum temperature above a threshold, which was 30°C for maize, 35°C for rice, 35°C for soy and 34°C for wheat, that are thought to damage crops. We used climate data provided by ISIMIP2a, and we extracted mean precipitation and EDDs for all grid cells within the country for which a drought or heatwave were reported.
For extreme temperature, we found that models with a higher EDD tended to better match yield decline found in observational data. But for precipitation we did not find a relationship between model performance and precipitation during simulated growing seasons. In further investigation we found a large variation between drought events. For some drought events the relationship was as expected (meaning that with low precipitation during the simulated growing season simulated yield decline was close to the observed yield decline), but for others, not. The latter was, for example, the case when there was large spatial heterogeneity within a country, where one part of the country experienced a drought while there was flooding in other parts of the country.
Limitations
There are three main limitations of this study. First, data quality of the observational yield data from the FAO database differs across countries. This is a source of uncertainty as a low agreement between observations and simulations does not necessarily mean that the model simulations are wrong, but could go back to errors in the observations. Second, extreme events are by definition rare, and the sample size is thus small, especially for heatwaves. In addition, we found that individual events can have a strong impact on the estimates of average observed yield decline, and events can impact only part of a country thus country-level aggregation introduces uncertainty. This aggregation is difficult to avoid because FAO data is reported at country-level. Lastly, how strongly an extreme event impacts crop yields is not only determined by the response of the plant, but also depends on how farmers respond, for example, by irrigation that can counteract the impact of high temperatures and droughts to some extent.
What this all means
Unfortunately, the main conclusion from this study is that climate change impacts on crop yields could be worse than currently estimated by global crop models. As the frequency and intensity of droughts and heatwaves is projected to increase further in the future, this could entail that crop yields are lower than currently estimated by crop models. Crop modellers are currently working on two aspects to improve model performance. First, they try to improve how heat stress and tissue damages under high temperatures are represented in the models. And secondly, there is ongoing work on improving the representation of growing seasons by including local observations. As the variation in crop yield is driven by weather only to a certain extent, combining crop models with models from other sectors that can include economic and social processes associated with extreme events (i.e., inter-sectoral modelling) could be an important step forward in the longer-term.
References
Cover image: Niki Clark
Affiliations
1 Potsdam Institute for Climate Impact Research